
In the first post in the Minimum Viable Data Stack series, we set up a process to start using SQL to analyze CSV data. You can even send custom dimensions from Google Sheets.ĪugSetting up Airbyte ETL: Minimum Viable Data Stack Part II
Postico run sql files how to#
I recently presented on how to get consistent metrics across Google Analytics, your ads platforms, and Hubspot called Marketing as a Data Product: Operational Analytics for Growth which shows how I did this. It feels a lot better to me than living and dying by button clicks. Sure the signal is imperfect (all models are) but it’s a lot stronger than the far-less-frequent conversion events. With reverse ETL data integration for Google Analytics, We can map these thresholds against custom dimensions to measure the volume of high-quality traffic a given channel is bringing in and evaluate channels/costs against traffic quality. With Clearbit Reveal and account-based scoring, we can put score-based thresholds on the traffic coming in (for example high/mid/low score). Firing a conversion based on an event gives us a really shallow view of success. But as you know, it was built for ecommerce-not for B2B SaaS. GA is the platform we trust for web analytics and a quick way to understand attribution. This is finally where Google Analytics comes in. So to advance our experimentation towards the top of the funnel, we need valid signals early in the journey. Growth is a process of expanding what works by experimenting and iterating.
Postico run sql files trial#
The problem is that those metrics are only helpful for understanding the funnel after a trial is started. Lead scores based on demographics, activity, content consumption, or product usage have been really helpful for us to aggregate signals into a few metrics that show how similar new leads are to past customers. It should come as no surprise that it’s easy for us to lose the connection between early marketing efforts and later sales outcomes. It’s a freemium product in the middle of multiple stakeholders and touchpoints. Here’s what I’m thinking.Ĭensus, as you’d imagine, has a long sales cycle. I could write a long list of game-changing applications for creating custom dimensions in Google Analytics from data in a Snoflake data warehouse but I’ve been swirling around one that I find particularly interesting. Last week, Census released our Google Analytics integration. Like many data geeks, Google Analytics was the thing that first sparked my curiosity. After a year of using it exclusively, I slowly found myself migrating back to psql and can honestly say I don't miss anything.īut it's also possible I just haven't tried the right GUI client yet.ApWriting Custom Dimension to Google Analytics from Snowflake DB I gave DataGrip an honest try to see what I might be missing from a GUI SQL client.
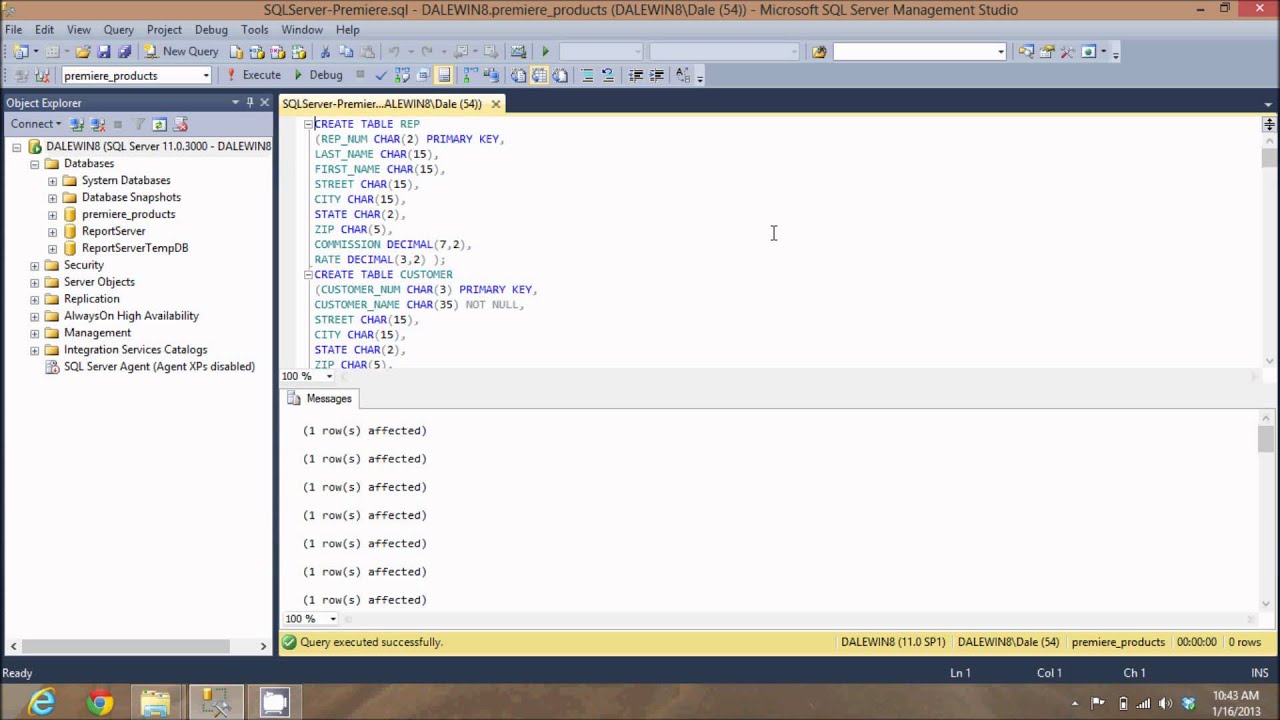
I used psql for a number of years, then joined a new company where DataGrip was the norm.

psqlrc file, like \x auto, and \timing and it becomes very usable very quickly.
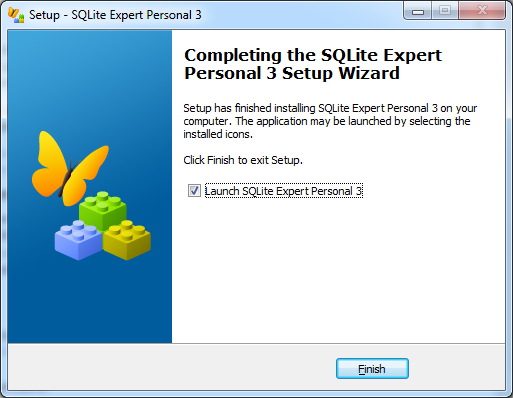
Take the time to customize it by adding a few lines to your. You can also nest additional \i my_other_query.sql commands inside the files you run with \i, which I've found really useful for repeatedly dropping / installing test tables / views, or setting up test data, before running the query I'm actually interested in. From the \d meta commands to inspect anything and everything in the database, to the variable substitution, nothing beats editing sql in your preferred editor and hitting \i my_query.sql in psql to run it.

Like Dimitri Fontaine says early in the Mastering PostgreSQL in Application Development book mentioned elsewhere in this thread, psql is really powerful. Other people have given actual answers, so I'll go ahead and give the non-answer of.
